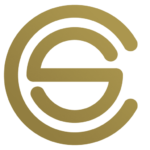
Adaptive control is a control theory that is designed to handle systems with unknown or time-varying parameters. The theory behind adaptive control involves the use of mathematical models to estimate the unknown parameters and to adjust the control inputs accordingly. There are several different types of adaptive control, including Lyapunov-based and MIT rule-based adaptive control.
Lyapunov-based adaptive control is a control method that uses the Lyapunov stability theory to ensure that the system remains stable even in the presence of unknown parameters. The Lyapunov function is used to derive an adaptive control law that adjusts the control inputs based on estimates of the unknown parameters. The advantage of Lyapunov-based adaptive control is its ability to handle systems with unknown or time-varying parameters while ensuring stability.
One of the disadvantages of Lyapunov-based adaptive control is that it can be difficult to design and tune the controller for complex systems. In addition, Lyapunov-based adaptive control can be sensitive to model inaccuracies and measurement noise, which can result in poor control performance.
MIT rule-based adaptive control is another type of adaptive control that uses a set of rules to adjust the control inputs based on estimates of the unknown parameters. The rules are designed based on the MIT rule-based adaptive control algorithm, which involves adjusting the control inputs based on the sign and magnitude of the estimated parameter error. The advantage of MIT rule-based adaptive control is its simplicity and ease of implementation.
One of the disadvantages of MIT rule-based adaptive control is its limited performance in handling complex systems. MIT rule-based adaptive control is typically used for systems with simple dynamics and low-order models. In addition, MIT rule-based adaptive control can be sensitive to noise and measurement inaccuracies, which can affect the accuracy of the estimated parameters and the control performance.
When deciding whether to use adaptive control instead of other control methods, the choice depends on the specific application. Adaptive control is well-suited for systems with unknown or time-varying parameters, where traditional control methods may not be effective. Adaptive control is particularly useful in applications where the parameters of the system are difficult to measure or are subject to change over time.
However, adaptive control can be more complex to design and tune than other control methods, and it can be sensitive to model inaccuracies and measurement noise. In addition, adaptive control can require more computational resources than other control methods, which can limit its applicability to real-time control applications.
In conclusion, adaptive control, including Lyapunov-based and MIT rule-based adaptive control, is a powerful control method that can handle systems with unknown or time-varying parameters. Adaptive control is particularly useful in applications where traditional control methods may not be effective. However, the choice of control method depends on the specific application requirements and limitations, and adaptive control can be more complex to design and tune than other control methods, and it can be sensitive to model inaccuracies and measurement noise.